UTS
Contents
UTS#
Lakukan analisa terhadap data pada https://archive.ics.uci.edu/ml/datasets/Breast+Cancer+Coimbra dengan menggunakan klasifikasi
metode KNN
metode pohon keputusan (Desision tree)
Proses analisa dilaporkan dan diupload di github ( menggunakan jupyter book)
Metode KNN#
Connect to google drive
Import Library
import pandas as pd
import numpy as np
import seaborn as sns
import matplotlib.pyplot as plt
Ambil data
%cd /content/drive/MyDrive/datamining/tugas/data/
/content/drive/MyDrive/datamining/tugas/data
data = pd.read_csv("dataR2.csv")
data.head()
Age | BMI | Glucose | Insulin | HOMA | Leptin | Adiponectin | Resistin | MCP.1 | Classification | |
---|---|---|---|---|---|---|---|---|---|---|
0 | 48 | 23.500000 | 70 | 2.707 | 0.467409 | 8.8071 | 9.702400 | 7.99585 | 417.114 | 1 |
1 | 83 | 20.690495 | 92 | 3.115 | 0.706897 | 8.8438 | 5.429285 | 4.06405 | 468.786 | 1 |
2 | 82 | 23.124670 | 91 | 4.498 | 1.009651 | 17.9393 | 22.432040 | 9.27715 | 554.697 | 1 |
3 | 68 | 21.367521 | 77 | 3.226 | 0.612725 | 9.8827 | 7.169560 | 12.76600 | 928.220 | 1 |
4 | 86 | 21.111111 | 92 | 3.549 | 0.805386 | 6.6994 | 4.819240 | 10.57635 | 773.920 | 1 |
Cek dataset
data
Age | BMI | Glucose | Insulin | HOMA | Leptin | Adiponectin | Resistin | MCP.1 | Classification | |
---|---|---|---|---|---|---|---|---|---|---|
0 | 48 | 23.500000 | 70 | 2.707 | 0.467409 | 8.8071 | 9.702400 | 7.99585 | 417.114 | 1 |
1 | 83 | 20.690495 | 92 | 3.115 | 0.706897 | 8.8438 | 5.429285 | 4.06405 | 468.786 | 1 |
2 | 82 | 23.124670 | 91 | 4.498 | 1.009651 | 17.9393 | 22.432040 | 9.27715 | 554.697 | 1 |
3 | 68 | 21.367521 | 77 | 3.226 | 0.612725 | 9.8827 | 7.169560 | 12.76600 | 928.220 | 1 |
4 | 86 | 21.111111 | 92 | 3.549 | 0.805386 | 6.6994 | 4.819240 | 10.57635 | 773.920 | 1 |
... | ... | ... | ... | ... | ... | ... | ... | ... | ... | ... |
111 | 45 | 26.850000 | 92 | 3.330 | 0.755688 | 54.6800 | 12.100000 | 10.96000 | 268.230 | 2 |
112 | 62 | 26.840000 | 100 | 4.530 | 1.117400 | 12.4500 | 21.420000 | 7.32000 | 330.160 | 2 |
113 | 65 | 32.050000 | 97 | 5.730 | 1.370998 | 61.4800 | 22.540000 | 10.33000 | 314.050 | 2 |
114 | 72 | 25.590000 | 82 | 2.820 | 0.570392 | 24.9600 | 33.750000 | 3.27000 | 392.460 | 2 |
115 | 86 | 27.180000 | 138 | 19.910 | 6.777364 | 90.2800 | 14.110000 | 4.35000 | 90.090 | 2 |
116 rows × 10 columns
print(len(data),len(data.columns))
116 10
Exploratory data analysis
data.info()
<class 'pandas.core.frame.DataFrame'>
RangeIndex: 116 entries, 0 to 115
Data columns (total 10 columns):
# Column Non-Null Count Dtype
--- ------ -------------- -----
0 Age 116 non-null int64
1 BMI 116 non-null float64
2 Glucose 116 non-null int64
3 Insulin 116 non-null float64
4 HOMA 116 non-null float64
5 Leptin 116 non-null float64
6 Adiponectin 116 non-null float64
7 Resistin 116 non-null float64
8 MCP.1 116 non-null float64
9 Classification 116 non-null int64
dtypes: float64(7), int64(3)
memory usage: 9.2 KB
Visualisasi correlation grid
plt.subplots(figsize=(20,20))
sns.heatmap(data.corr(),cmap='RdYlGn',annot=True)
<matplotlib.axes._subplots.AxesSubplot at 0x7f46426c4e10>
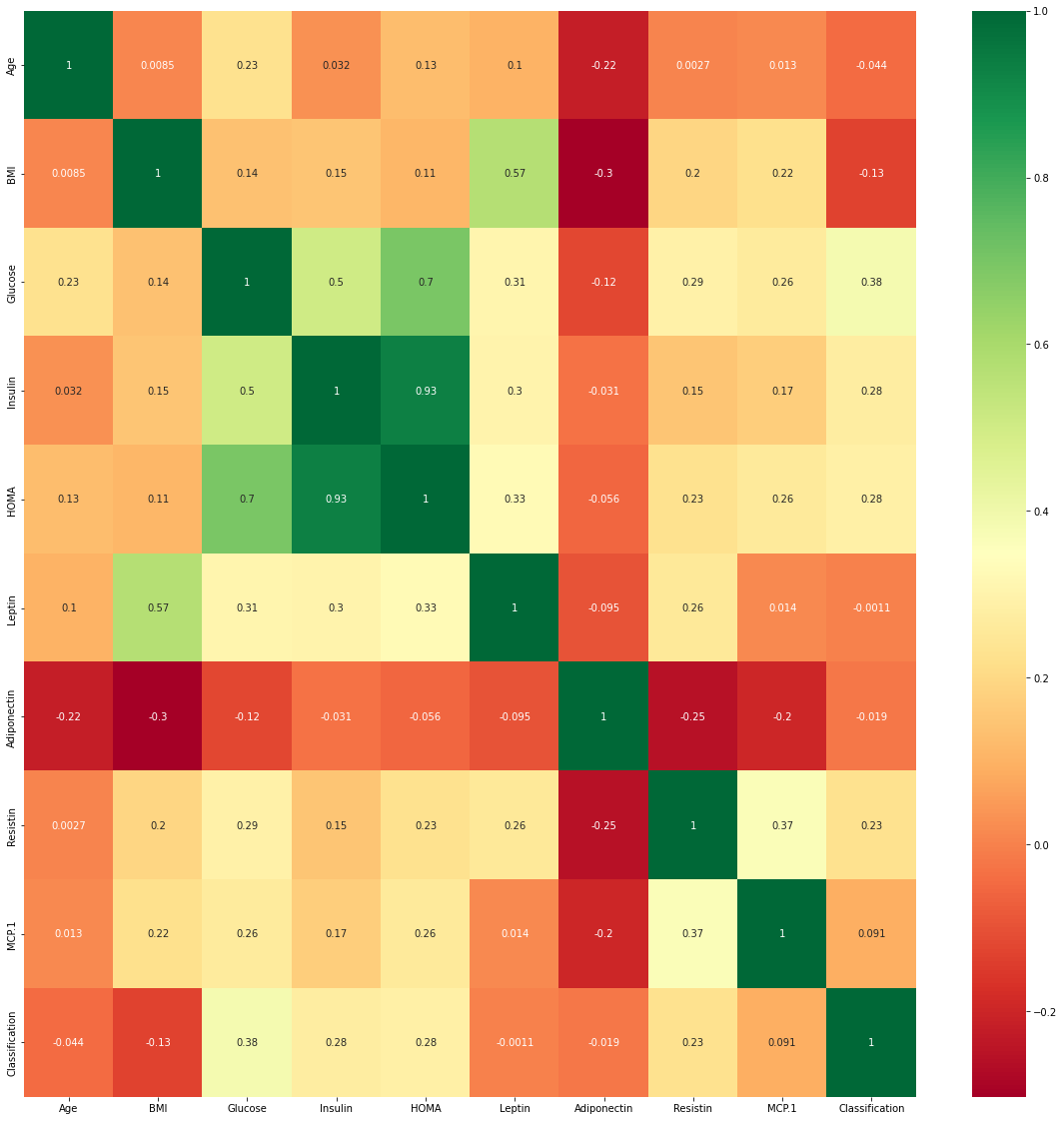
# create the features from data
X=data.iloc[:,0:9]
# create the target variable from data
Y=data.iloc[:,9]
X.head()
Age | BMI | Glucose | Insulin | HOMA | Leptin | Adiponectin | Resistin | MCP.1 | |
---|---|---|---|---|---|---|---|---|---|
0 | 48 | 23.500000 | 70 | 2.707 | 0.467409 | 8.8071 | 9.702400 | 7.99585 | 417.114 |
1 | 83 | 20.690495 | 92 | 3.115 | 0.706897 | 8.8438 | 5.429285 | 4.06405 | 468.786 |
2 | 82 | 23.124670 | 91 | 4.498 | 1.009651 | 17.9393 | 22.432040 | 9.27715 | 554.697 |
3 | 68 | 21.367521 | 77 | 3.226 | 0.612725 | 9.8827 | 7.169560 | 12.76600 | 928.220 |
4 | 86 | 21.111111 | 92 | 3.549 | 0.805386 | 6.6994 | 4.819240 | 10.57635 | 773.920 |
Y.head()
0 1
1 1
2 1
3 1
4 1
Name: Classification, dtype: int64
Menggunakan Standardisasi untuk membawa semua nilai ke satu unit karena KNN adalah metode berbasis jarak
from sklearn.preprocessing import StandardScaler
ss=StandardScaler()
X=ss.fit_transform(X)
X=pd.DataFrame(X)
Splitting of Dataset untuk uji
from sklearn.model_selection import train_test_split
xtrain,xtest,ytrain,ytest=train_test_split(X,Y,test_size=0.3)
Membangun classifier KNeighbors menggunakan simulasi nilai k yang berbeda
#Importing KNeighbors Classifier from sklearn
#Finding accuracies on TrainData and Test data with euclidean distance(by default p=2)
from sklearn.neighbors import KNeighborsClassifier
from sklearn.metrics import accuracy_score
for x in range(5,10,2):
knn=KNeighborsClassifier(n_neighbors=x,metric='minkowski',weights='distance')
knn.fit(xtrain,ytrain)
train_ypred=knn.predict(xtrain)
acc_train_score=accuracy_score(train_ypred,ytrain)
test_ypred=knn.predict(xtest)
acc_test_score=accuracy_score(test_ypred,ytest)
print(f'Skor akurasi untuk data uji adalah {acc_train_score} and {acc_test_score} untuk {x} neighbours')
Skor akurasi untuk data uji adalah 1.0 and 0.8285714285714286 untuk 5 neighbours
Skor akurasi untuk data uji adalah 1.0 and 0.7714285714285715 untuk 7 neighbours
Skor akurasi untuk data uji adalah 1.0 and 0.6857142857142857 untuk 9 neighbours
Membangun classifier KNeighbors menggunakan Eucledian Distance dan 7 neighbours
knn=KNeighborsClassifier(n_neighbors=7,metric='minkowski',weights='distance')
knn.fit(xtrain,ytrain)
KNeighborsClassifier(n_neighbors=7, weights='distance')
# Predicting for train data
trainypred=knn.predict(xtrain)
Finding the precision, recall, f1-score ,support
# Classification report on train data set
from sklearn.metrics import classification_report
print(classification_report(trainypred,ytrain))
precision recall f1-score support
1 1.00 1.00 1.00 36
2 1.00 1.00 1.00 45
accuracy 1.00 81
macro avg 1.00 1.00 1.00 81
weighted avg 1.00 1.00 1.00 81
# Mencari skor akurasi
accuracy_score(trainypred,ytrain)
1.0
Memprediksi data tes
testypredicted=knn.predict(xtest)
# Skor akurasi data tes
from sklearn.metrics import accuracy_score
accuracy_score(testypredicted,ytest)
0.7714285714285715
Metode Decision Tree#
Classification
# classifying
from sklearn.tree import DecisionTreeClassifier
from sklearn.model_selection import train_test_split
from sklearn.metrics import accuracy_score
from io import StringIO
# pretty printing
from pprint import pprint
# visualizing
import matplotlib.pyplot as plt
from IPython.display import Image
from sklearn.tree import export_graphviz
import pydotplus
from sklearn.datasets import load_breast_cancer
data = load_breast_cancer()
# The shape of the data matrix (without class attribute)
print("Matrix shape: " + repr(data.data.shape))
# The names of the features
print("The data set has the following features:")
pprint(data.feature_names)
# The names of the classes
print("The data set has the following classes:")
pprint(data.target_names)
pprint(data.data[1])
# This prints a rather long descriptions.
# print(data.DESCR)
Matrix shape: (569, 30)
The data set has the following features:
array(['mean radius', 'mean texture', 'mean perimeter', 'mean area',
'mean smoothness', 'mean compactness', 'mean concavity',
'mean concave points', 'mean symmetry', 'mean fractal dimension',
'radius error', 'texture error', 'perimeter error', 'area error',
'smoothness error', 'compactness error', 'concavity error',
'concave points error', 'symmetry error',
'fractal dimension error', 'worst radius', 'worst texture',
'worst perimeter', 'worst area', 'worst smoothness',
'worst compactness', 'worst concavity', 'worst concave points',
'worst symmetry', 'worst fractal dimension'], dtype='<U23')
The data set has the following classes:
array(['malignant', 'benign'], dtype='<U9')
array([2.057e+01, 1.777e+01, 1.329e+02, 1.326e+03, 8.474e-02, 7.864e-02,
8.690e-02, 7.017e-02, 1.812e-01, 5.667e-02, 5.435e-01, 7.339e-01,
3.398e+00, 7.408e+01, 5.225e-03, 1.308e-02, 1.860e-02, 1.340e-02,
1.389e-02, 3.532e-03, 2.499e+01, 2.341e+01, 1.588e+02, 1.956e+03,
1.238e-01, 1.866e-01, 2.416e-01, 1.860e-01, 2.750e-01, 8.902e-02])
# Split into training and test
X_train, X_test, y_train, y_test = train_test_split(data.data[:,0:9],data.target,shuffle=True,test_size=0.3, random_state=42)
# DECISION TREE
# initialize the model with standard parameters
clf_dt = DecisionTreeClassifier(criterion="entropy")
# train the model
clf_dt.fit(X_train,y_train)
DecisionTreeClassifier(criterion='entropy')
# Evaluating on the test data
y_test_pred = clf_dt.predict(X_test);
a_dt_test = accuracy_score(y_test, y_test_pred);
# Evaluating on the training data
y_train_pred = clf_dt.predict(X_train);
a_dt_train = accuracy_score(y_train, y_train_pred);
print("Training data accuracy is " + repr(a_dt_train) + " and test data accuracy is " + repr(a_dt_test))
Training data accuracy is 1.0 and test data accuracy is 0.9590643274853801
dot_data = StringIO()
export_graphviz(clf_dt, out_file=dot_data,
feature_names=data.feature_names[0:9],
class_names=data.target_names,
filled=True, rounded=True,
special_characters=True)
graph = pydotplus.graph_from_dot_data(dot_data.getvalue())
Image(graph.create_png())
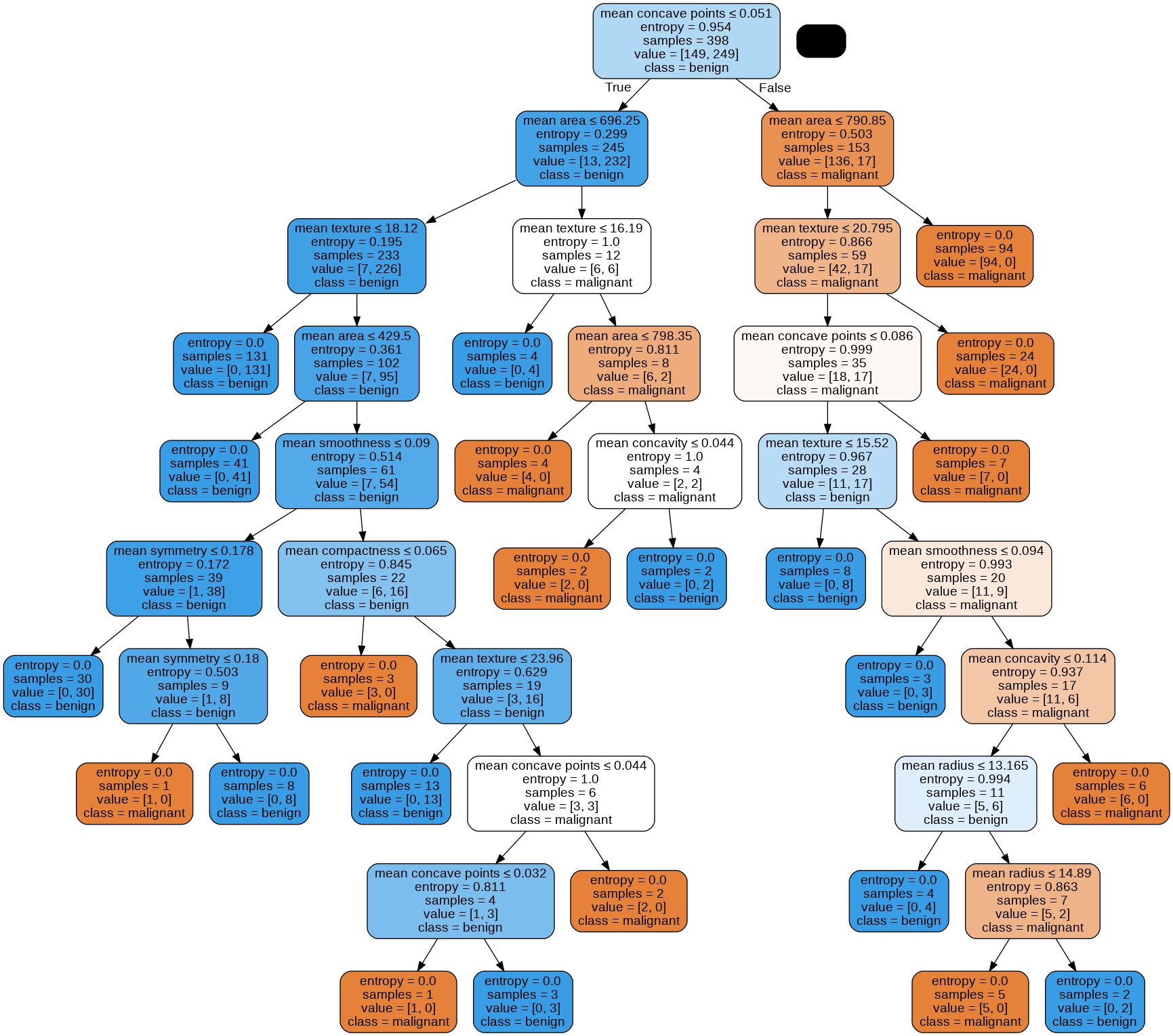